A second research line is visual
attention and the respective
electrocortical correlates. I'm especially interested in the
question whether EEG parameters reflect dimension based processes
as it was demonstrated by Muller and colleagues (1995, 1996). Based on
their 'Dimension Weighting Account' we conducted several experiments
using EEG recordings to to uncover the temporal dynamics of weight
shifting on consecutive trials.
In a first series of experiments we showed that in visual search, there
is a reaction time (RT) cost for targets on a given trial if the
previous target was defined in a different dimension. According to the
'dimension-weighting account' (Muller, Heller, & Ziegler, 1995),
limited attentional weight needs to be shifted to the new dimension,
resulting in slower RTs. We aimed at identifying brain electrical
correlates associated with the weight shift. Analyses of ERPs revealed
several components to reflect dimension changes whether the task was to
detect the target or to identify its defining dimension. N2 amplitudes
were more negative whenever the dimension changed. The P3 exhibited
latency differences that mirrored RTs in both tasks, but the amplitudes
showed no direct relation to stimulus- or response-related processes.
Finally, slow-wave amplitudes were enhanced for dimension changes.
Taken together, the results provide support for relatively early,
perceptual processes underlying dimension change costs (Gramann,
Toellner, Krummenacher, Eimer & Muller (2007), Psychophysiology).
In a second experimental approach, we tried to dissociate perceptual
and motoric influences on dimension-based weighting mechanisms. By
focusing on the N2pc and the lateralized readines potential (LRP) we
were able to demonstrate, that
in
cross-dimensional visual search tasks, target discrimination is
expedited when the previous trial contained a target defined in the
same visual dimension as the current trial. The DWA explains this
RT-pattern by
assuming that visual dimensions are weighted on a perceptual stage of
processing. Recently, this view has been challenged by models in
which the allocation of attentional resources is assumed to occur
after visual encoding mechanisms (Mortier et al., 2005). To
disentangle whether the intertrial facilitation effect is due to a
“perceptual” stage, “response selection“ stage, or a
combination of both stages, we recorded EEG during a compound task.
Participants first had to search for a singleton target, defined by a
unique dimension (colour or shape), before the appropriate response,
which was independently determined by the orientation of the target,
could be selected. Visual dimension repetitions were mirrored by
shorter latencies and enhanced amplitudes of the N2pc suggesting a
facilitated allocation of attentional resources to the actual target.
Response repetitions and changes systematically modulated the LRP
amplitude suggesting a benefit from residual activations of the
previous trial biasing the correct response. Overall, the present
findings strengthen the DWA indicating a perceptual origin of
dimension change costs in visual search (Toellner, Gramann, Muller,
Kiss & Eimer (2008), Journal of Experimental Psychology: Human
Perception and Performance).
Lately, we focussed on the influence of dimensional weighting on early
sensory processes as reflected in the visual evoked P1-component. The
selection of targets in a visual scene can be
based on positional information or non-spatial features operating in
a location-independent manner. Here we
investigated whether dimension-based attention effects (i) can be
observed for early visual information processing
and
(ii) whether the number of possible target locations in visual search
influences dimension based processes. To test this, a visual
search task for singletons with non-predictive featural but
predictive locational trial-by-trial cueing
(Experiment
1), or non-predictive dimensional and non-predictive locational
identity of the upcoming target (Experiment 2) was conducted. The
results revealed systematic dimension-based variations of the early
visual evoked P1 in both experiments.
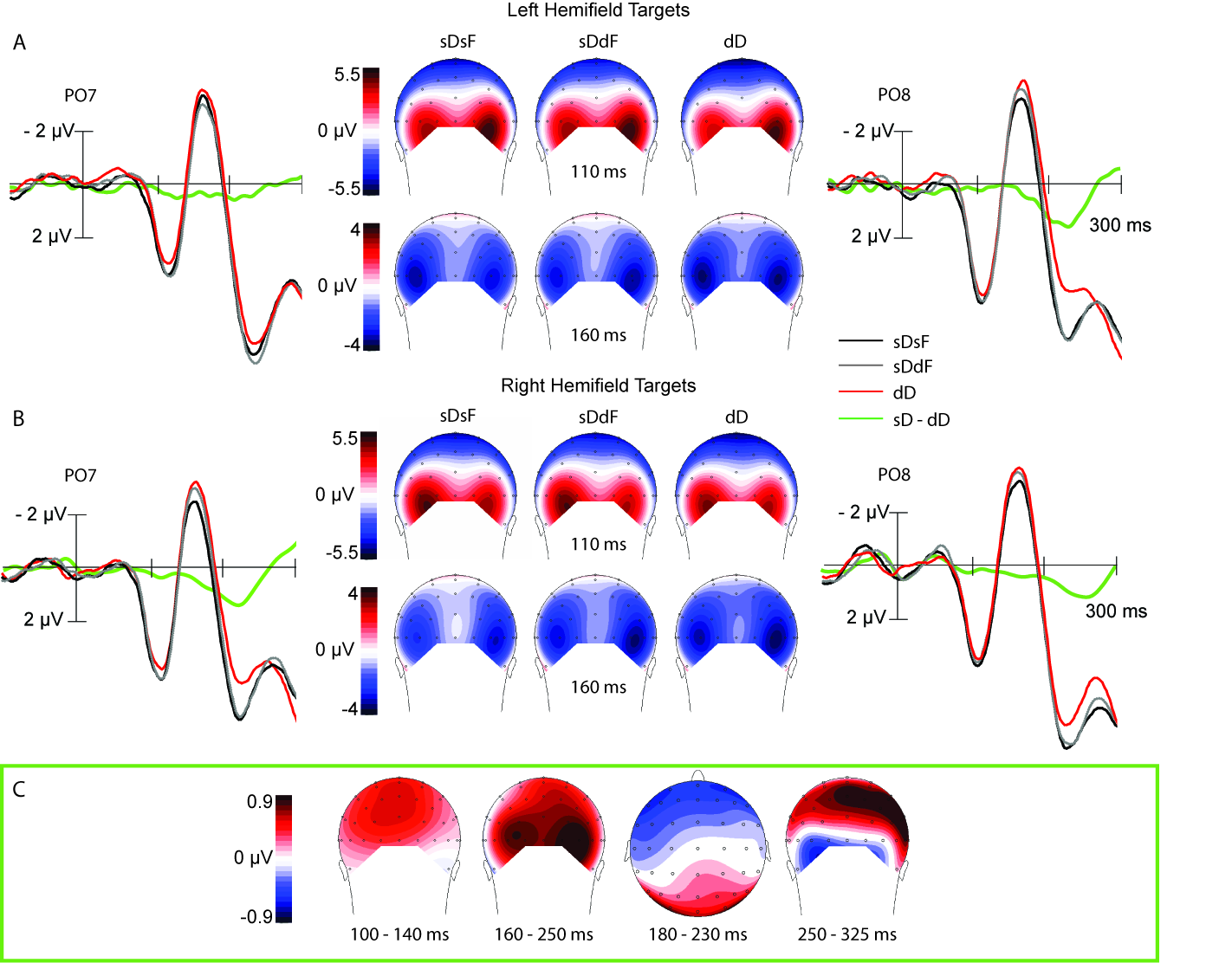
Figure 1: Grand-average ERP waveforms eklicited over early
visual areas, recorded at electrode positions PO7/PO8 contra- and
ipsilateral to (A) left hemifield targets and (B) right hemifield
targets in a 300 ms intervall following stimulus onset. Black lines
represent feature repetitions between the cue and target singletons
(sDsF), dark grey lines intra-dimensional feature changes (sDdF), and
red lines dimension changes (dD). The middle column displays scalp maps
for the visual evoked P1 component (upper row) and the N1 component
(lower row) for the 3 experimental conditions. (C) represents scalp
maps for difference waveforms, computed by subtracting different
dimension trials from same dimension trials. Scalp maps are displayed
for 4 different time windows.
Figure 2: Spatio-temporal coupled density reconstruction for
same- and different dimension trials. Left and right columns display
current souorce activation for the visual P1 and tN2 component,
for (a) same dimension same feature trials (sDsF), (B) same dimension
diferent feature trials (sDdF), and (C) different dimension trials
(dD). Last row (D) displays regions revealing significant dimension
based modulations on source level.
This effect was independent of
the featural identity within the cued dimension. In addition, the
anterior transition N2 (tN2) was increased for dimension changes
relative to repetitions. Based on these components, source
reconstructions demonstrated dimension change-related activations in
left frontopolar and dorsal occipital cortex. The dimension-based
non-spatial influence on early visual processing is in line with
dimension-based theories on visual attention (e.g. DWA) and provides
evidence for the processing of dimensional information as early as
110 ms post-stimulus (Gramann, K., Toellner, T., & Muller, H.,
2009, Psychophysiology).
Using a data-driven analysis approach, we extended the results and
demonstrated a network of frontal independent component clusters to
modulate the surface recorded activity (Onton, J., Toellner, T.,
Mueller, H., & Gramann, K., in preparation).
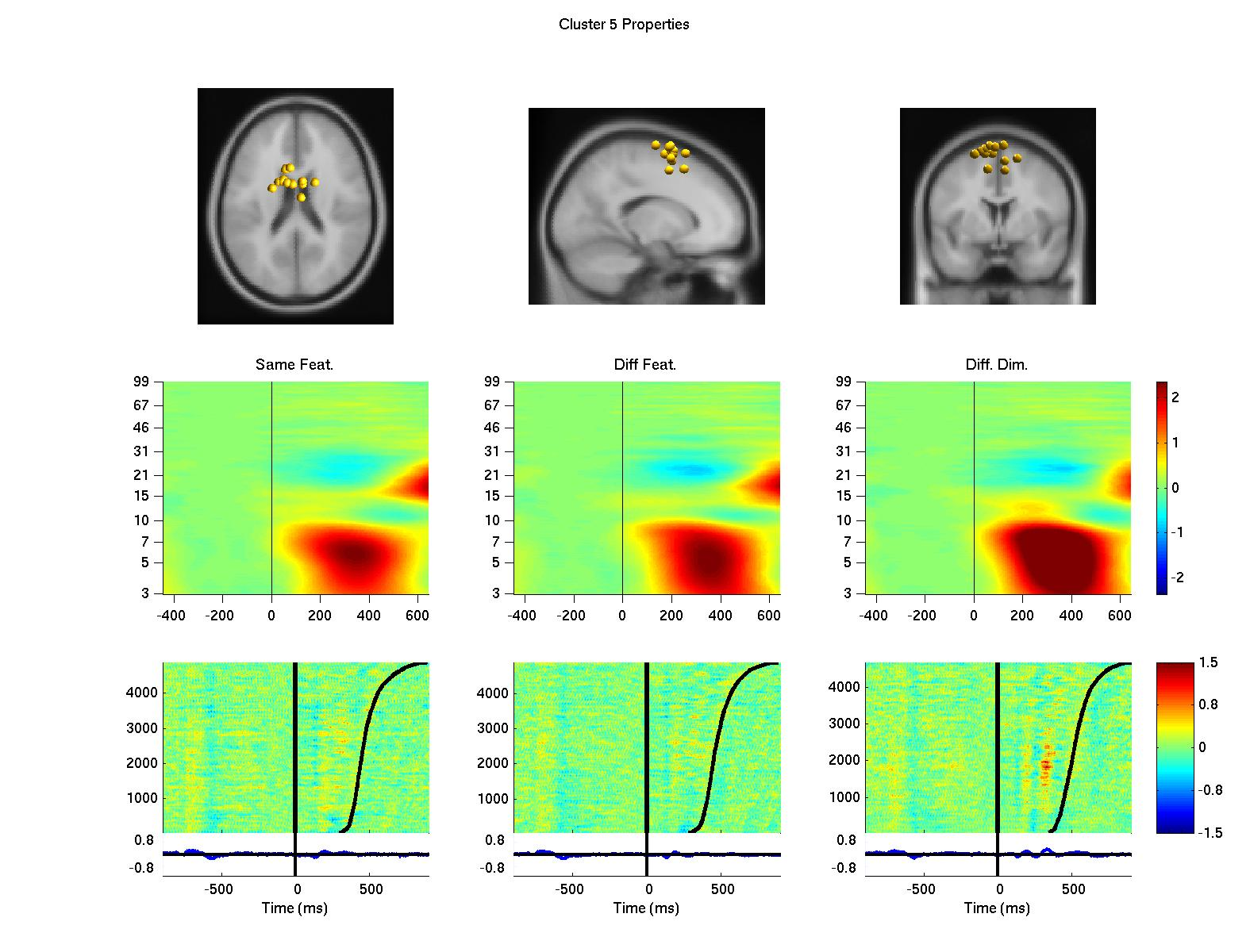
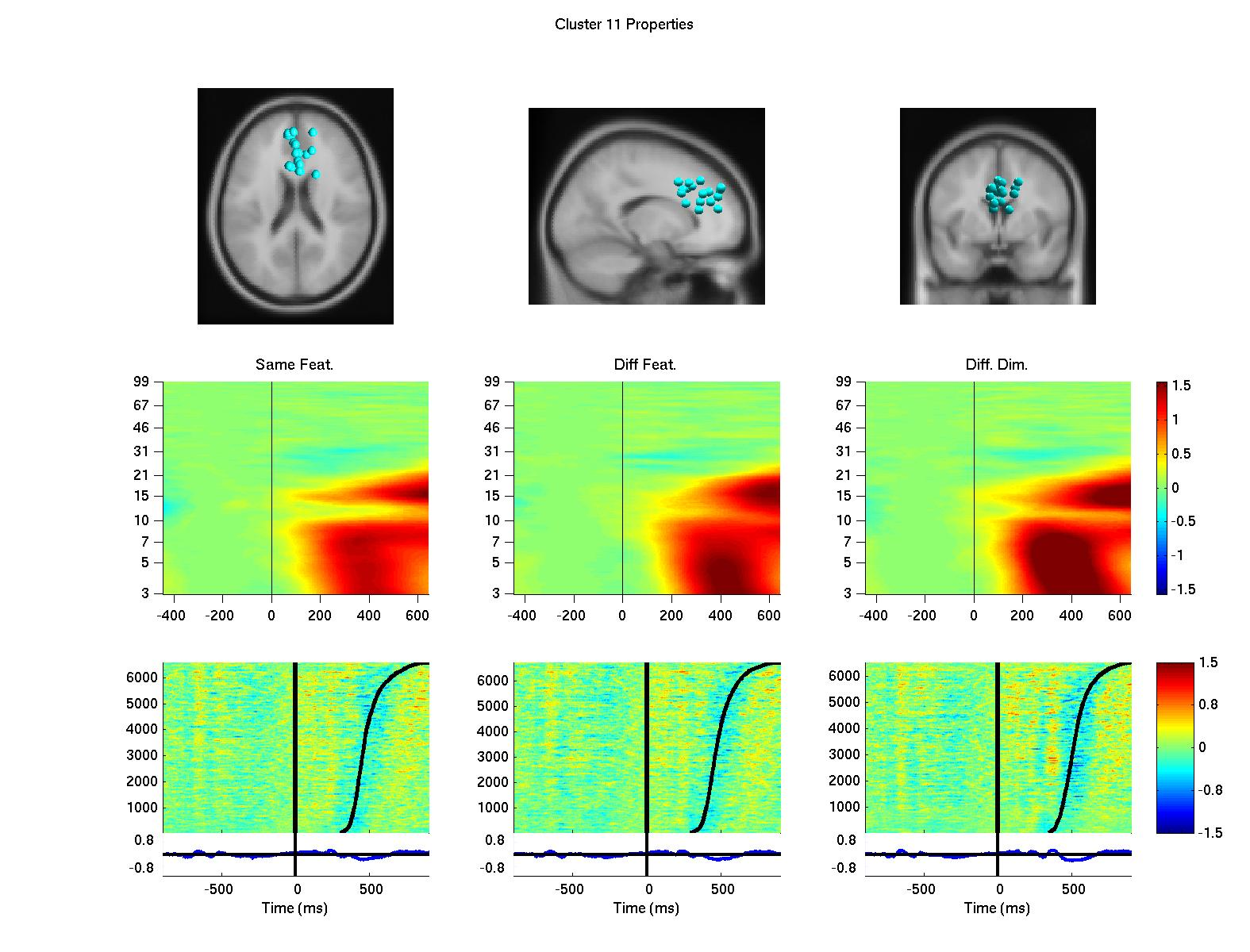
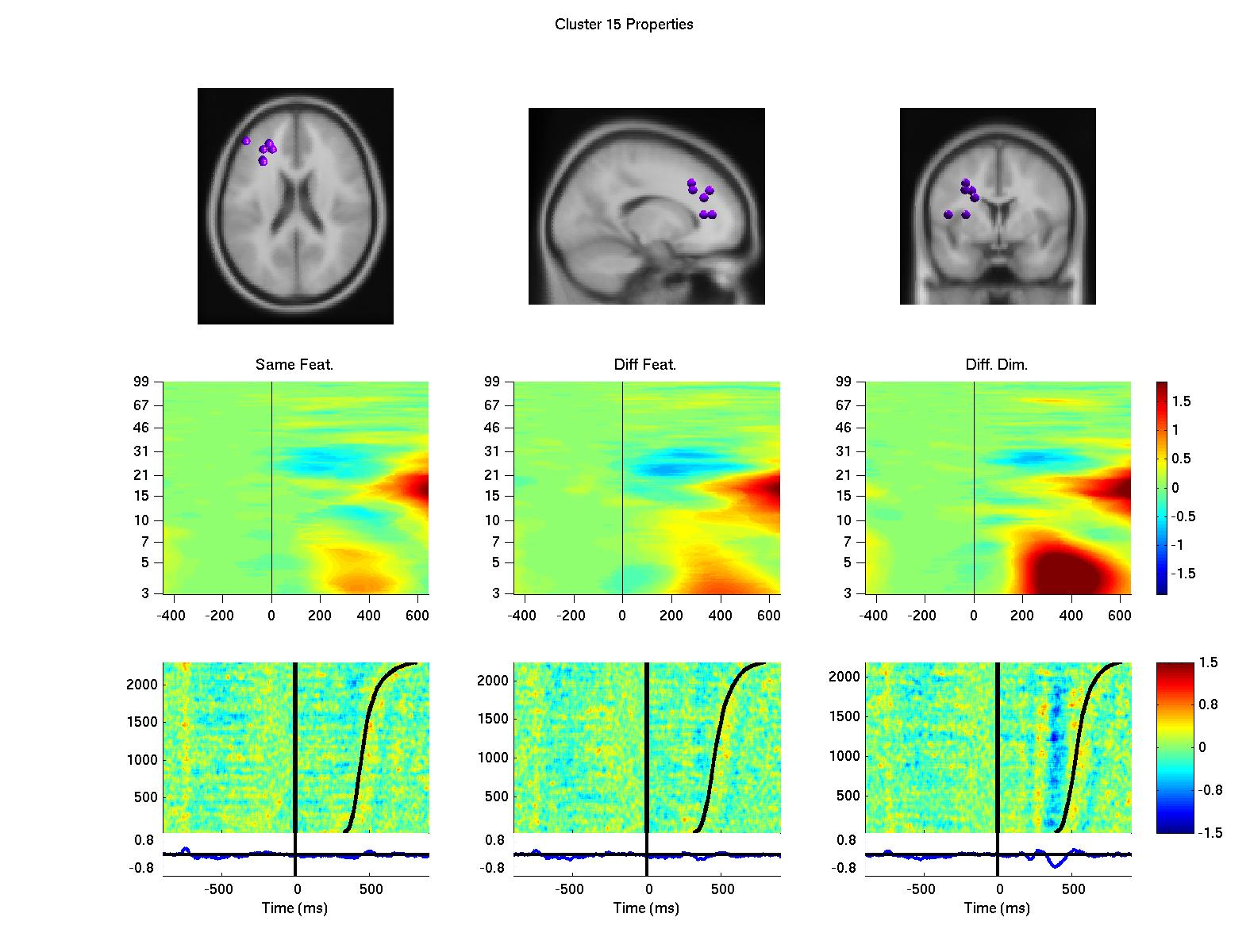
Figure 2: Clusters of independent component processes with
cluster centroids (first row) located in or near the premotor cortex
(left column), the anterior cingulate cortex (second from left), the
left prefrontal cortex (third from left) and the right prefrontal
cortex (right column). Clusters were computed using K-means algorithm
based on baseline spectrum, event-related spectral perturbation,
intertrial coherence, event-related potentials, scalp maps, and dipole
locations. The second row displays event-related spectral perturbation
of compnent clusters for same dimension same feature trials, same
diemnsion different feature trials, and different dimension trials.
Last row represents erp-images sorted by reaction times (second black
line). The first black line indicates onset of the target display.