The EEGLAB News #8
Fiorenzo Artoni, Ph.D. (continued)
Click here to return to main profile
Research Topics
Artificial Intelligence: This line of Dr. Artoni's research regards algorithms for decoding brain signals to predict, for example, intention of movement. Recently he and his colleagues validated a Long-Short-Term Memory (LSTM) deep learning model to decode gait phases from Electroencephalography (EEG) and demonstrated how the use of hybrid EEG and EMG interfaces can increase the decoding stability of Brain Computer Interfaces (Tortora et al., 2020a; Tortora et al., 2020b).
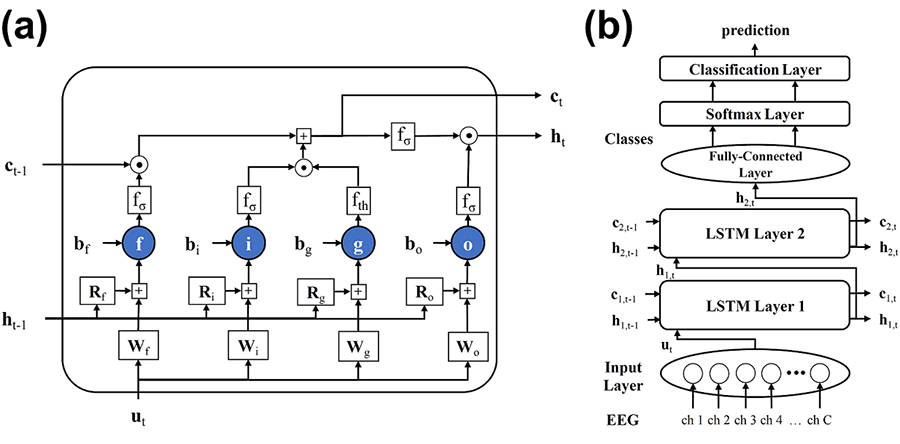
Schematics of a Long-Short-Term Memory (LSTM) deep learning model,
that was successfully used to decode and predict gait phases from EEG activity
collected during steady-state walking (Tortora et al., 2020a).
As well, he and colleagues proposed a novel shared control scheme in which robotic automation aids in object grasping by maximizing the contact area between the hand and the object, thus greatly increasing grasp success and object hold times in both a virtual and a physical environment. These results presented a viable method of prosthesis control implemented in real time, for reliable articulation of multiple simultaneous degrees of freedom.
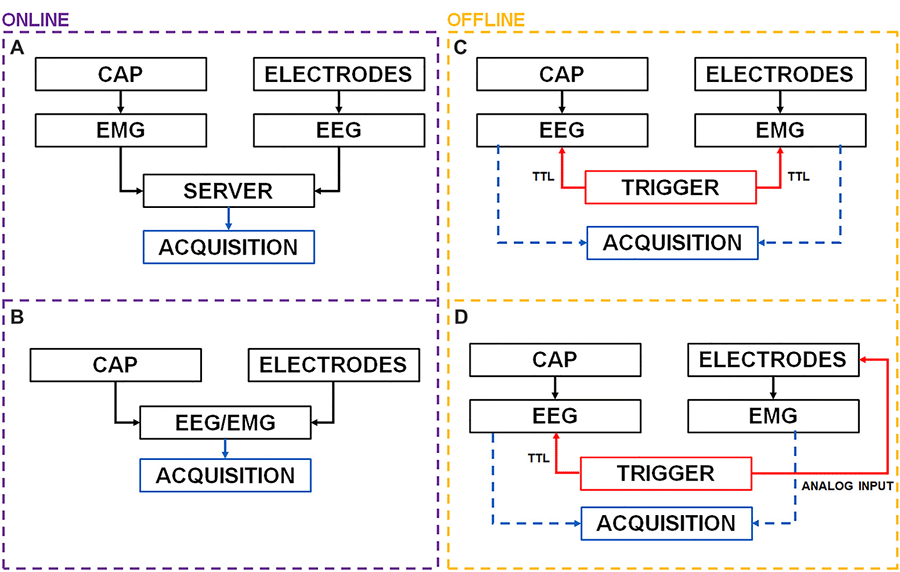
Scheme representing different strategies for offline and online synchronization
of multiple streams of data for MoBI experiments, both offline (C,D)
and online (A,B) (Artoni et al., 2016)
Data Science. This line of his research regards the development of algorithms to successfully disentangle information from noise and estimate the neuro-biomechanical state of the patient in near real time. This requires:
- the development of tools for EEG processing that allow an efficient removal of EEG artifacts (Artoni et al., 2012),
- the development of tools for the unified analysis of EEG and EMG (Artoni et al., 2016),
- to determine how best to extract muscle synergies during gait (Artoni et al., 2013),
- to explore novel connectivity methods based on direct Directed Transfer Function (dDTF) to estimate brain-to-muscle directional connectivity (as opposed to non-directional coherence) that is specific, informative and time-locked to gait phases (Artoni et al., 2017)
- to estimate the reliability of Independent Components (RELICA) (Artoni et al., 2014).
Mobile Brain/Body Imaging. Mobile Brain/body Imaging (MoBI) is a promising emerging field of research and can be defined as “an integrative multimethod approach used to investigate human brain activity, motor behavior, and other physiological data associated with cognitive processes that involve active behavior”. MoBI opens the doors to many research areas including cognitive control of locomotion, brain-body interactions in neurological disorders and Brain Machine Interfaces (BMIs). For this reason, he is developing the tools to assemble a modular, high-resolution, real-time, non-invasive, whole-body neuroimaging Mobile Brain-Body Imaging platform, able with minimum effort from the user to synchronize, collect, integrate, store and analyze physiological measures such as EEG, electromyographic (EMG), kinematics and ground-reaction-force (GRF) data that is able to provide clinicians with reliable real-time cortico-cortical and cortico-muscular connectivity measures and other useful information for the assessment of the neuro-biomechanical state of the patient. (Figure - Left panel: Scalp map and localization of sources that are active in rhythm with the gait cycle. Right panel: Brain-to-Muscle connectivity during walking (swing phase), each line connecting a specific region of interest in the brain to one muscle. Artoni et al., 2017).
Cited references:
Artoni, F., Menicucci, D., Delorme, A., Makeig, S., Micera, S. (2014). RELICA: a method for estimating the reliability of independent components. Neuroimage, 103, December 2014, 391-400.
Martínez-Cancino, R., Delorme, A., Truong, D., Artoni, F., Kreutz-Delgado, K., Sivagnanam, S., Yoshimoto, K., Majumdar, A., Makeig, S. (2020). The Open EEGLAB Portal Interface: High-Performance Computing with EEGLAB. Neuroimage, 116778.
Artoni, F., Delorme, A., Makeig, S. (2018). Applying dimension reduction to EEG data by Principal Component Analysis reduces the quality of its subsequent Independent Component decomposition. NeuroImage.
Artoni, F., Fanciullacci, C., Bertolucci, F., Panarese, A., Makeig, S., Micera, S., Chisari, C. (2017). Unidirectional brain to muscle connectivity reveals motor cortex control of leg muscles during stereotyped walking. Neuroimage 159, 403-416.
Other references:
Artoni, F., Chisari, C., Menicucci, D., Fanciullacci, C., Micera, S. (2012). REMOV: EEG artifacts removal methods during Lokomat lower-limb rehabilitation. Biomedical Robotics and Biomechatronics (BioRob), 2012 4th IEEE RAS & EMBS International Conference on, pp. 992-997.
Artoni, F., Fanciullacci, C., Bertolucci, F., Panarese, A., Makeig, S., Micera, S., Chisari, C. (2017). Unidirectional brain to muscle connectivity reveals motor cortex control of leg muscles during stereotyped walking. Neuroimage, 159, 403-416.
Artoni, F., Menicucci, D., Delorme, A., Makeig, S., Micera, S. (2014). RELICA: a method for estimating the reliability of independent components. Neuroimage, 103, December 2014, 391-400.
Artoni, F., Monaco, V., Micera, S. (2013). Selecting the best number of synergies in gait: preliminary results on young and elderly people. IEEE Int Conf Rehabil Robot 2013, 6650416.
Artoni, F., Pirondini, E., Panarese, A., Micera, S. (2016). Exploring Neuro-Muscular Synergies of Reaching Movements with Unified Independent Component Analysis. IEEE EMBC 2016.
Genna, C., Oddo, C., Fanciullacci, C., Chisari, C., Micera, S., Artoni, F. (2018). Bilateral cortical representation of tactile roughness. Brain research 1699, 79-88.
D’anna, E., Petrini, F.M., Artoni, F., Popovic, I., Simanić, I., Raspopovic, S., Micera, S. (2017). A somatotopic bidirectional hand prosthesis with transcutaneous electrical nerve stimulation based sensory feedback. Scientific reports 7, 10930.
Martínez-Cancino, R., Delorme, A., Truong, D., Artoni, F., Kreutz-Delgado, K., Sivagnanam, S., Yoshimoto, K., Majumdar, A., Makeig, S. (2020). The Open EEGLAB Portal Interface: High-Performance Computing with EEGLAB. Neuroimage, 116778.
Tortora, S., Ghidoni, S., Chisari, C., Micera, S., Artoni, F. (2020a). Deep learning-based BCI for gait decoding from EEG with LSTM recurrent neural network. Journal of neural engineering. Volume 17, Number 4.
Tortora, S., Tonin, L., Chisari, C., Micera, S., Menegatti, E., Artoni, F. (2020b). Hybrid Human-Machine Interface for Gait Decoding Through Bayesian Fusion of EEG and EMG Classifiers. Frontiers in Neurorobotics, 14, 89.